Predicting Pitches
- Bruin Sports Analytics
- Dec 16, 2022
- 7 min read
By: Alisha Dhar

The MLB has been the leading sport to utilize analytics in recent years. From deciding where to place fielders in a shift to predicting which prospects are going to be successful in the major leagues, data analytics is incorporated into all aspects of the game. A few years ago, the Houston Astros were caught using a camera system in game to steal signs which were relayed to batters. While this was clearly a violation of rules and a blatant act of immorality, pitch prediction using analytics morally is still a huge asset to MLB teams and a lineup’s ability to succeed off of pitchers. So, is it possible to predict pitches?
This article will take a look at the use of different pitches in different scenarios and attempt to determine patterns in discerning which pitch will be thrown next. In each visualization the following key will apply.
FF = four-seam fastball
SL = slider
FT = two-seam fastball
CH = changeup
SI = sinker
CU = curveball
FC = cutter.
For simplicity, other pitches (eephus, forkball, knuckle ball, etc. ) have been removed from the plots and analysis since they rarely appear in comparison to the seven pitches listed above.
First, let’s look at a general graph of pitches thrown regardless of scenario.
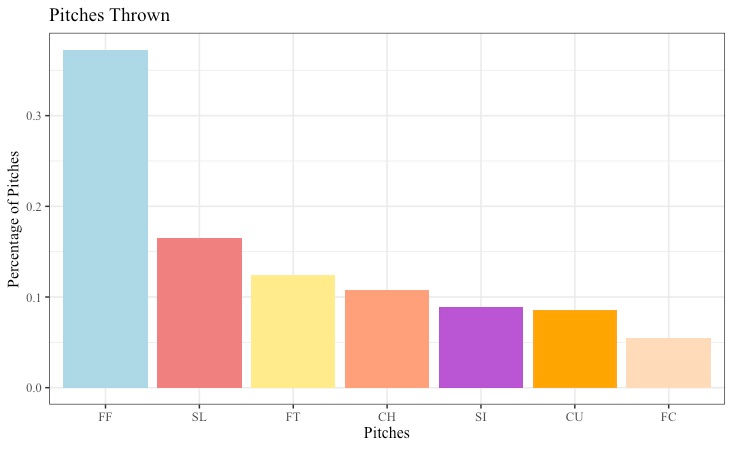
As expected, four-seam fastballs are thrown the most of all pitches – approximately 35.40%. The next most common pitch is the slider at 15.72%, followed by two-seam fastballs at 11.79% and changeups at 10.21%. Sinkers and curveballs are both thrown at similar rates: around 8.46% and 8.18% of the time respectively. Thrown the least are cutters at just over 5%. This can be used as a baseline when looking into the percentages of pitches thrown in different counts, which are graphed next.
Pitches Thrown in Each Count
Although these bar graphs show similar rank in frequency of pitch type thrown to the plot depicting pitches thrown regardless of count, we can use these graphs to determine when certain pitches are thrown more or less than normal.
For example, four-seam fastballs, although consistently thrown more than all other pitches, are thrown at a 10% greater rate (around 45% of the time) in situations with 3 balls. Because this is a “strike-needed” scenario (whereas in other counts pitchers have a strike to give), pitchers tend to go for a four-seam fastball because it has the most predictable movement.
In this three-ball scenario, we can also see that the two-seam fastball is the second most pitch thrown while the slider is the second most pitch thrown in every other count. This is for a similar reason – although two-seam fastballs have more movement than four-seam fastballs, they still tend to have less movement than offspeed pitches.
Another note is that curveballs are thrown with the fourth highest frequency in 0-0, 0-1, and 0-2 counts and with the lowest frequency in 3-0, 3-1, and 3-2 counts. This is the biggest range in rank of all the pitches and can be explained by the unpredictable nature of the curveball. The curveball is known to have the greatest movement of all pitches in baseball and is also one of the most difficult to face. Two of the most unhittable pitches in MLB history are known to be Clayton Kershaw and Sandy Koufax’s curveballs, which have a 12-6 drop. This means that the baseball starts at the top of or above the strike zone (where the 12-hand is on a clock) and drops vertically below the zone (where the 6-hand is on a clock). Many pitchers also have horizontal movement in their curve (making a 2-8 or 10-4 break). Because of this movement, it is more difficult for pitchers to control curveballs and guarantee a strike, so it is used in situations where pitchers have a ball to give and may want to make the batters chase a ball. This explains its greater use in low counts where the pitcher is ahead and low use in counts with three balls.
Changeups are also thrown at a greater frequency in 1-0, 1-1, and 1-2. While this is difficult to explain given that a 1-0 count is a very different scenario than a 1-2 count, future analysis could look at the location where this pitch is thrown in these different counts. It is expected that a 1-0 changeup would be more in the zone while a 1-2 changeup would be more of a chase pitch.
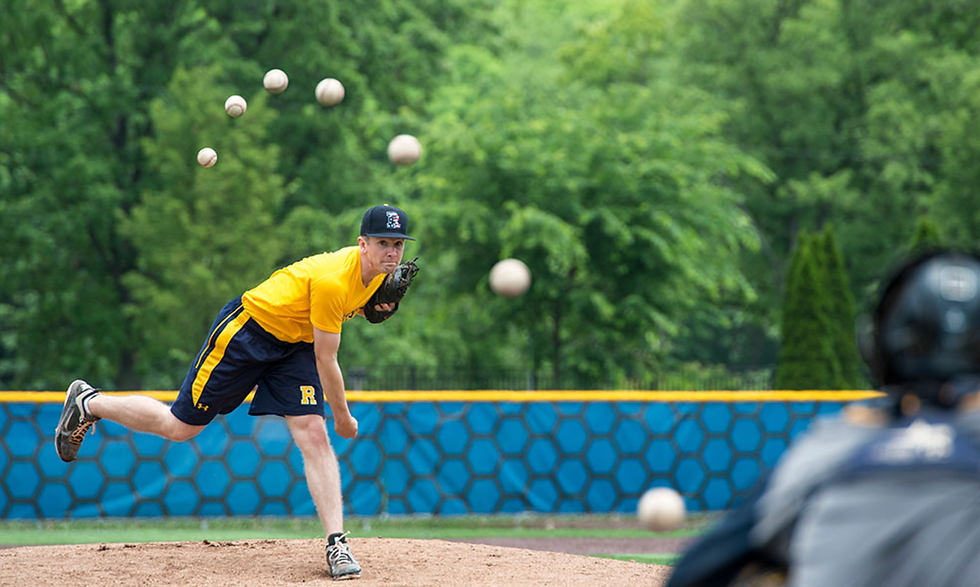
Utilizing the Previous Pitch Sequence
Given the number of potential sequences for predicting pitches, I have chosen to focus on a few specific scenarios to analyze.
Changeup
First, we will look at the pitches thrown after a changeup, dividing changeups thrown for a strike and changeups thrown for a ball.
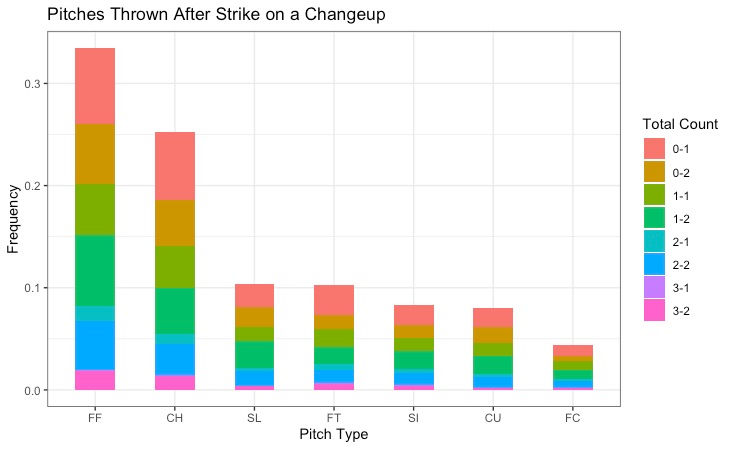
Following a strike on a changeup, the most commonly thrown pitch is a four-seam fastball at around 33%. This is similar to the overall percentage of four-seam fastballs per pitches thrown, and is not surprising since changeups can be used to throw off the batter before changing speeds again. Changeups are the second most commonly thrown pitch in this scenario, thrown around 25% of the time. This differs drastically from the general percentage of changeups thrown (10%). Given that this scenario only looks at changeups where the batter swung and missed, fouled off the pitch, or took a strike, it is likely that the batters were fooled by the offspeed pitch – meaning that the pitcher could take advantage of this and try to fool them with another offspeed. Interestingly, we also see a high percentage of changeups after a changeup is thrown for a ball (see graph below). One note though, is that the graph above shows the two highest counts for this second changeup are 0-1 and 0-2, while the graph below shows the two highest counts for the second consecutive changeup are 1-0 and 1-1. This shows that pitchers are more confident in throwing a second changeup when they are not too behind in the count, regardless of the previous pitch outcome.
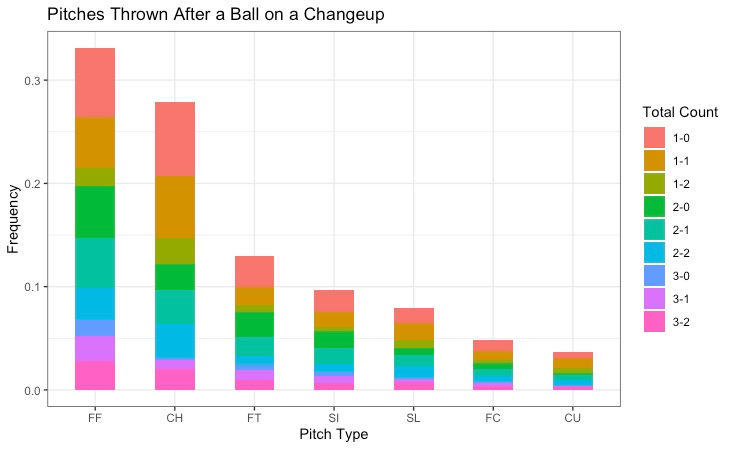
Foul Ball
We can next look at the situations following a foul ball, comparing foul balls off of fastballs and offspeed pitches.
From these graphs, we can see that both four-seam fastballs and two-seam fastballs are thrown at a greater rate following a fastball fouled off versus after an offspeed pitch fouled off. Four-seam fastballs are thrown 41.6% of the time in the first graph versus 30.2% of the time in the second graph, and two-seam fastballs are thrown 12.2% of the time in the first graph versus 7.4% of the time in the second graph. The difference in curves thrown, surprisingly, is similar to that of the two-seam fastball: after fastballs, it is 11.0%, but after offspeed pitches it is 7.8%. This indicates a nearly opposite strategy from throwing a two-seam fastball in these situations. The pitch with the biggest change, however, is the sinker. While it is thrown just 1.5% of the time after a fastball is fouled, it is thrown 17.0% of the time after an offspeed pitch is fouled off. Unlike the two other faster pitches, it is utilized more after the offspeed in this scenario; this could indicate that pitchers are trying to force a swing and miss or a weak ground ball in play after a batter was able to make contact in the previous pitch. One overarching note is that there is a greater range in the frequency of pitches thrown following a fastball fouled (40.1%) versus an offspeed pitch fouled (23.3%), indicating that it is more difficult to predict the following pitch after an offspeed pitch is fouled off.
Runners in Scoring Position
This final plot shows pitches thrown when there are runners in scoring position.

Interestingly, there is not a notable difference in the proportion of pitches thrown in the graph above (where we filter by only runners on second and/or third base) and the general Pitches Thrown graph (the first graph in the article). One point is that there is a more even split in the count each pitch is thrown – in previous graphs, there was greater variation. For example, certain pitches were thrown more in certain counts than others, but in this scenario with runners in scoring positions, each pitch is thrown pretty evenly across all counts. One future step to find a more clear pattern in this situation could be to divide this by right-handed and left-handed pitchers and hitters. Since pitchers are frequently trying to force the batter to hit the ball on the left side of the field (towards the third baseman and shortstop) in order to prevent a run from scoring, different pitches would force this with different right-hand/left-hand pairings of pitchers and hitters.
Conclusions and Future Work
While it does seem possible to find trends and patterns in which pitch is thrown in various scenarios, the number of different factors that can change pitch by pitch make it difficult to pinpoint a certain pitch. For example, different pitchers have different strengths, and different batters have different weaknesses. Even going into the specifics of how many runners are on base, the score, the inning, the batter’s recent hitting performance, which ballpark the game is being played in, whether the fielders are playing in a shift, etc. have an influence on the next pitch thrown.
In the future, one thing I would like to do is look more into pitch sequences – looking at the likelihoods of combinations of at least two pitches together and using these to predict the next pitch. I would also like to find more specific scenarios that correspond to critical or unique situations in baseball; for example, close-scoring games in the later innings with runners on base, or the first at-bat after a home run, well-hit ball, or run scored. It would also be interesting to apply this topic to specific pitchers or hitters. I would also like to figure out how to apply machine learning to this pitch prediction scenario to create a model that can determine pitch sequencing and account for a larger range of factors.
Source:
MLB Pitch Data 2015-2018 on Kaggle
Comments