By: Hashim Bhat
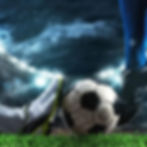
Introduction
The English Premier League is the most watched soccer league in the world, boasting a global audience of 3.2 billion people worldwide. The overwhelming majority of fans who support a team in the EPL root for one of the “Big 6” and, every year, they bite their nails as they hope to reach for those top 4 champions league spots, or even contend for the Premier League title. An overlooked aspect of the league, though no less of a nail-biter, is the battle that goes on at the bottom of the table, where teams fight to stay out of the relegation zone. When it comes to this battle, newly promoted teams from the second division (the EFL Championship) often face the most pressure due to the huge increase in the level of competition they face. Incentivized by the fact that they stand to bring in up to hundreds of millions more in revenue by playing in the Premier League as compared to the Championship, these teams have plenty to play for. However, transitioning from being a dominant force in the 2nd division, to avoiding being considered an “easy 3 points” in the 1st division is no easy task. It is not uncommon that a newly promoted team only lasts a single season in the Premier League before being relegated back to the second division. But we have also seen cases where these presumed underdogs have managed to tussle their way up to the middle of the pack, outperforming all expectations and showing up to those who doubted them. This begs the question: what causes these newly promoted teams to be more, or less, successful in their 1st Premier League season? This article aims to answer this question using data.
Background
The EFL Championship is the second tier of English soccer. At the end of every season, the top 2 teams are automatically granted promotion to the Premier League, while teams ranked 3rd to 6th compete in a playoff tournament where the winner gets the third promotion spot.
The Premier League is the 1st tier of English soccer, and at the end of every season, the bottom 3 teams in the league (the teams ranked 18th to 20th) are relegated to the EFL Championship.
Definitions
Null Hypothesis: The null hypothesis is the claim that no relationship exists between the variables that we are examining. So if we reject the null hypothesis, it would follow that a relationship does in fact exist. Rejecting the null hypothesis is the same as accepting the “alternative hypothesis”, which is the claim that a certain relationship does exist (opposite of the null hypothesis).
P-value: A p-value is the probability of obtaining results at least as extreme as the result that was actually observed. Hence it follows that a low p-value means that we should reject a null hypothesis, because it is statistically very unlikely to observe our results which implies that some kind of relationship or correlation must exist.
Significance Level: If we got a p-value of 0.07, we would reject the null hypothesis at a significance level of 0.10 (also known as a 10% significance level) because our p-value is lower than the significance level. Since the sample sizes that we are working with in this article are small, I will use a 10% significance level.
Methodology
Using Python, I worked with spreadsheets that included data pertaining to every fixture from a given season in the EFL Championship. For multiple seasons, for the 3 teams that won promotion that season, I created tables displaying the variables defined below:
Predictor Variables
Average Home Goals: Average number of goals scored at home fixtures in the EFL Championship the season that promotion was achieved
Average Home Conceded: Average number of goals conceded at home fixtures in the EFL Championship the season that promotion was achieved
Average Away Goals: Average number of goals scored at away fixtures in the EFL Championship the season that promotion was achieved
Average Away Conceded: Average number of goals conceded at away fixtures in the EFL Championship the season that promotion was achieved
Experience in the Premier League: How many seasons the team has played in the English Premier League since it was founded in 1993
Club expenditure: How much money a team spent on players in the summer transfer window in their 1st Premier League season following promotion.
Predicted Variable
EPL Ranking: Ranking that a team achieved in their 1st Premier League season. Because a lower ranking indicates a better performance, I defined this as the number of places from last place, e.g. if they ranked 20th, I assigned a ranking of 20-20 = 0, if they ranked 19th, I assigned a ranking of 20-19 = 1.
Using all of this information, I created a dataframe that looks like this:

I read this dataframe into R and did some regression analysis to find any patterns or correlations between the predictor variables and the EPL Ranking.
The first step of regression analysis is always to make some simple visualizations to get a better idea of relationships between the different variables. So I obtained the following scatterplots for each predictor variable vs EPL Ranking:
The strongest trend from these plots is the positive association between EPL Ranking and club expenditure. EPL Ranking may also be positively associated with Average Home Goals Scored. Plots by themselves only give us a general idea of any associations there may be, but we must run a regression model to see if these predictor variables are actually statistically significant towards explaining the EPL Ranking.
So I created a multiple regression model using all of the predictor variables. The p-value of the multiple regression model itself was 0.309. This suggests that, at the 10% significance level, none of our predictor variables are statistically significant. However, the p-value of Club Expenditure was 0.116 and the p-value of Average Home Goals was 0.121 which are both close to being significant at the 10% significance level. So I made a reduced model, which means that I removed all other predictor variables apart from Club Expenditure and Average Home Goals to see how this affects our results. In multiple regression, we are accounting for all of the variables involved as we try to determine significance, therefore making a new model where we remove variables that are definitely unrelated can give us a better, more accurate model. The reduced model had a p-value of 0.067, which means that one of our predictor variables is statistically significant and is correlated with EPL Ranking. The individual p-value of Club Expenditure was 0.087 and the p-value of Average Home Goals was 0.195. Hence, it follows that, out of all of our predictor variables, club expenditure was the only one that was statistically significant.
Conclusion and Limitations
The regression analysis showed us that the amount of money that a newly promoted side spends in the summer transfer window on players has a statistically significant positive effect on the ranking of that team in the Premier League the following season.
Interestingly, none of the variables related to the teams performance in the Championship during the season of promotion turned out to be statistically significant at the 10% significance level, although the amount of goals scored at home could possibly have a weakly positive relationship.
This goes towards showing the huge gap in skill and quality demanded between the 2nd and 1st division of english football. Just because a team performs well in a certain metric in the championship, it does not mean that this will continue in the Premier League because the opposing teams are so much stronger.
It is important to note that the sample size of our model was only 32 which is very small for a multiple regression analysis model, so the findings of our model should be taken with a grain of salt. Adding more observations and having more data would definitely improve the accuracy of our findings and could perhaps also lead to some new findings in regards to associations between the variables.
If you wanted to predict the exact ranking of newly promoted teams in the Premier League, I don’t think that any of our predictor variables, including club expenditure, would enable you to do that accurately because there is too much uncertainty and too many factors at play . That being said, if you specifically want to predict whether a newly promoted team will avoid relegation in its first Premier League season, your best bet is to look at how much money that team spends in the summer transfer window, because spending big is what seems to consistently enable these teams to perform better according to statistical analysis.
Sources: